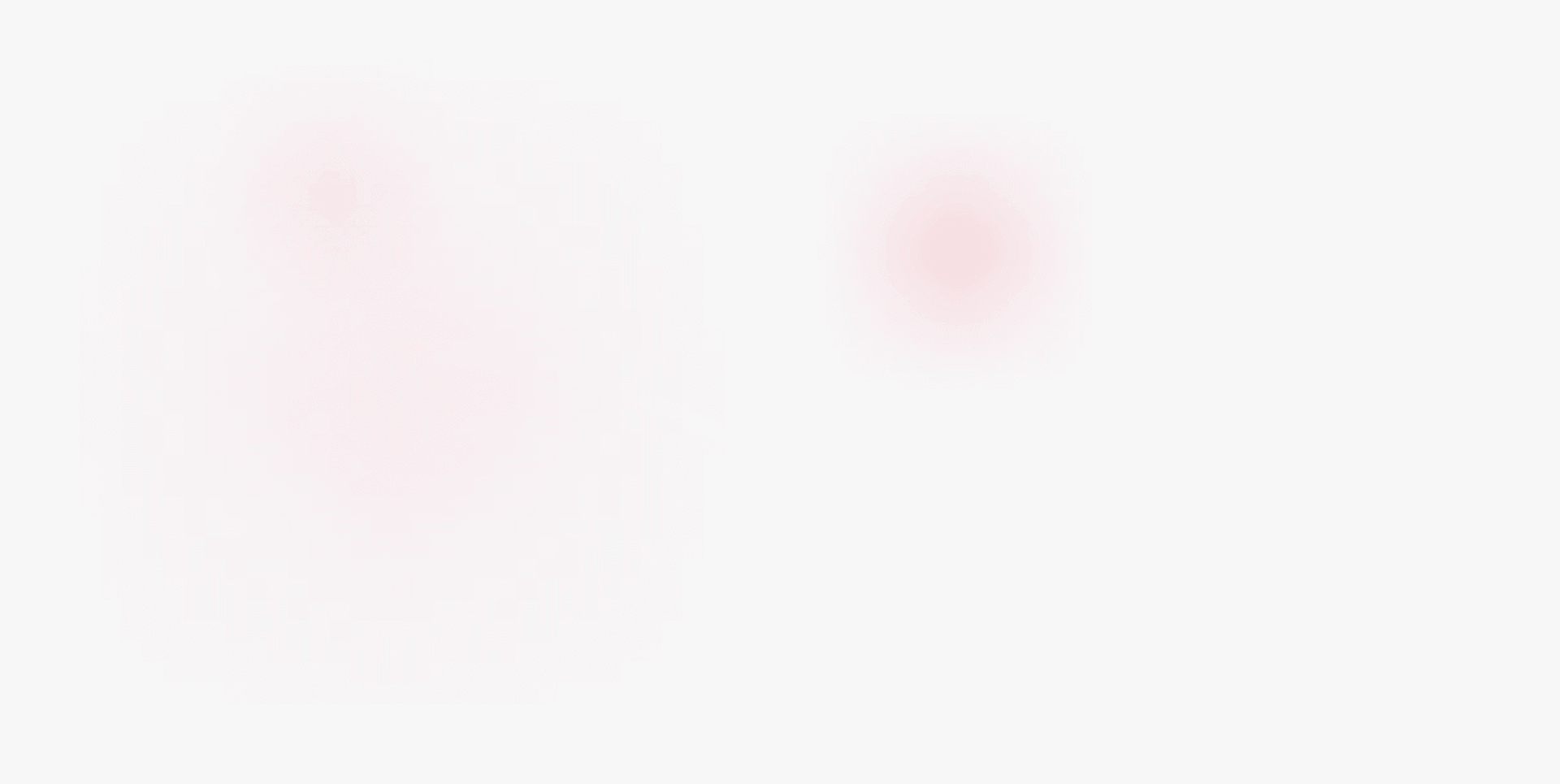
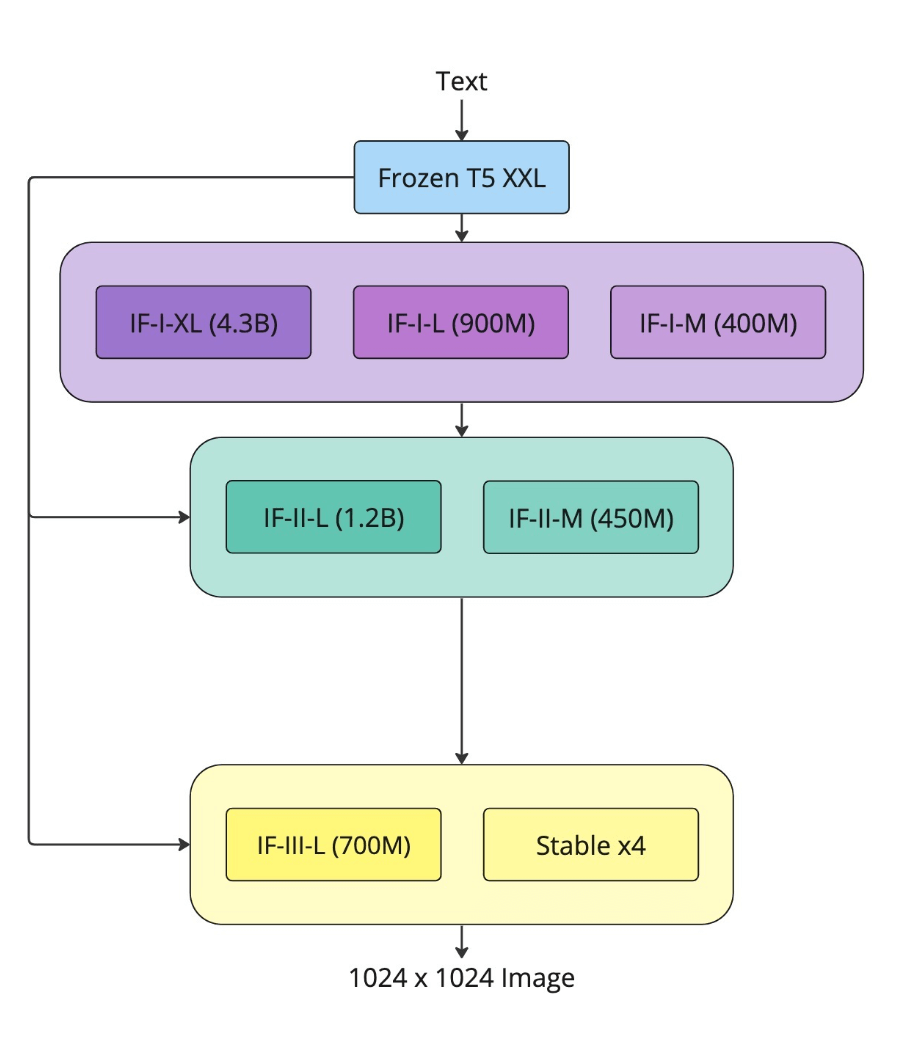
T2I Models Explained,
DeepFloyd
DeepFloyd-IF is an advanced text-to-image diffusion model that excels in both photorealism and language comprehension. It achieves impressive results, surpassing existing models in terms of efficiency and performance. Specifically, it achieves a remarkable zero-shot FID-30K score of 6.66 on the COCO dataset. DeepFloyd-IF is designed as a modular system, consisting of a frozen text mode and three pixel cascaded diffusion modules. These modules generate images at progressively higher resolutions: 64x64, 256x256, and 1024x1024. The model employs a frozen text encoder, based on the T5 transformer, to extract text embeddings. These embeddings are then utilized in a UNet architecture, which incorporates cross-attention and attention-pooling techniques for enhanced image generation.
Model Card

100+ Technical Experts

50 Custom AI projects

4.8 Minimum Rating
Blockchain Success Starts here


-
About Model
-
Model Highlighter
-
Training Details
-
Evaluation Results
-
Usage
-
Sample Codes
-
Other LLMs
About Model
The researchers introduce DeepFloyd IF, an advanced open-source text-to-image model known for its impressive photorealism and language understanding. DeepFloyd IF is designed as a modular system consisting of a text encoder that remains unchanged and three-pixel diffusion modules that work in a cascaded manner. The base model generates 64x64 px images based on the provided text prompt. It is then followed by two super-resolution models designed to generate images of increasing resolution: 256x256 px and 1024x1024 px.
The entire model utilizes a frozen text encoder based on the T5 transformer. This encoder extracts text embeddings, which are then passed through a UNet architecture enhanced with cross-attention and attention pooling. Combining these components results in an efficient model surpassing existing state-of-the-art models. It achieves a remarkable zero-shot FID score of 6.66 on the COCO dataset, highlighting its exceptional performance.
The findings of this research emphasize the potential of utilizing larger UNet architectures in the initial stage of cascaded diffusion models. It also provides a glimpse into a promising future for text-to-image synthesis.
Developed by: DeepFloyd Lab at StabilityAI
License: DeepFloyd IF License Agreement
Model Highlights
The IF-4.3B base model is the largest diffusion model in terms of the number of effective parameters of the U-Net. It achieves a state-of-the-art zero-shot FID score of 6.66, outperforming both Imagen and the diffusion model with expert denoisers eDiff-I. A deep text understanding is achieved by employing a large language model T5-XXL as a text encoder, using optimal attention pooling, and utilizing the additional attention layers in super-resolution modules to extract information from the text.
- IF is designed with a collaborative approach, incorporating multiple neural modules that work together within a single architecture to achieve a synergistic effect. The model follows a cascading approach to generate high-resolution images. It starts with a base model that produces low-resolution samples, further enhanced by a series of upscale models to create visually stunning, high-resolution images.
- The base model and the super-resolution models in IF utilize diffusion models, which employ Markov chain steps to introduce random noise into the data. This process is then reversed to generate new data samples from the noise, resulting in improved image quality. Unlike latent diffusion techniques like Stable Diffusion, which rely on latent image representations, IF operates directly within the pixel space. This approach allows for more precise manipulation and generation of images.
- Transitioning from the shadows to the light, image-to-image translation can now be accomplished through a simple yet effective process. By resizing the original image to 64 pixels and introducing a controlled amount of noise using forward diffusion, followed by denoising the image with a fresh prompt during the backward diffusion process, remarkable transformations can be achieved.
- IF demonstrates a remarkable affinity for text, skillfully incorporating it into various artistic mediums. Whether it's embroidering text onto fabric, integrating it into a stained-glass window, including it in a collage, or illuminating it on a neon sign, IF excels in these challenging text-to-image scenarios. Previous text-to-image models have faced difficulties in achieving such versatility, making IF a pioneering solution in this regard.
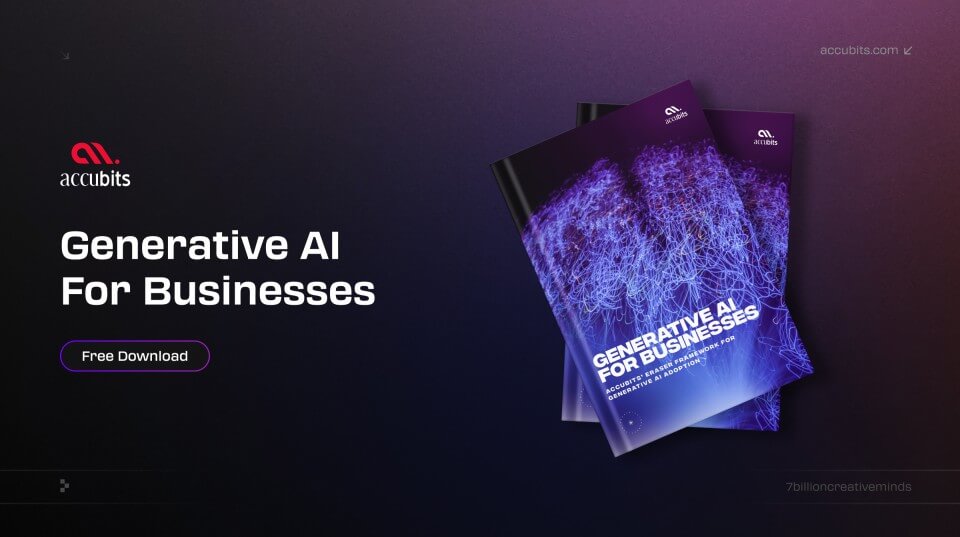
Training Details
The model is trained on 1.2B text-image pairs (based on LAION-A and few additional internal datasets). Test/Valid parts of datasets are not used at any cascade and stage of training. Valid part of COCO helps to demonstrate "online" loss behaviour during training (to catch incident and other problems), but dataset is never used for train.
Training Procedure
During the training phase, the images undergo preprocessing steps. First, they are cropped to a square shape using shifted-center-crop augmentation, where the cropping position is randomly shifted up to 0.1 of the image size. The cropped images are then resized to 64 pixels using the BICUBIC resampling method provided by Pillow version 9.2.0. To avoid aliasing, the reducing_gap=None parameter is utilized. The processed images are converted into a tensor format with dimensions BxCxHxW.
Text prompts play a crucial role in the model's training. They are encoded using a pre-trained frozen T5-v1_1-xxl text-encoder developed by the Google team. Approximately 10% of the texts are intentionally dropped to an empty string as part of the training process. This technique, known as classifier-free guidance (CFG), adds flexibility to the model's ability to interpret and generate diverse outputs.
The non-pooled output of the text encoder undergoes further processing. It is fed into a projection layer, which is a linear layer without any activation function. This output is then utilized in the UNet backbone of the diffusion model, incorporating controlled hybrid self- and cross-attention mechanisms. Additionally, the output of the text encoder is pooled using attention-pooling with 64 heads. This pooled output is further utilized in time embedding as additional features.
The diffusion process is performed through 1000 discrete steps, employing a cosine beta schedule for noising the image. This schedule helps in gradually introducing noise into the image throughout the diffusion process.
The loss function employed during training is a reconstruction objective, comparing the noise added to the image with the prediction generated by the UNet.
For the specific IF-I-XL-v1.0 checkpoint, the training process comprises 2,420,000 steps at a resolution of 64x64 across all datasets. The training utilizes the OneCycleLR policy, few-bit backward GELU activations, and employs the AdamW8bit optimizer in combination with DeepSpeed-Zero1. The T5-Encoder remains fully frozen during the training process.
Training Settings
The model was trained using a powerful hardware setup consisting of 64 x 8 x A100 GPUs. The optimization process utilized the AdamW8bit optimizer in combination with DeepSpeed ZeRO-1. Each training batch consisted of 3072 samples. The learning rate was scheduled using the one-cycle cosine strategy, with a warmup period of 10000 steps. The initial learning rate (start_lr) was set to 2e-6, reaching a maximum learning rate (max_lr) of 5e-5, and finally settling at a final learning rate (final_lr) of 5e-9.
Evaluation Results
FID score of 6.66 was observed as the training evaluation result.
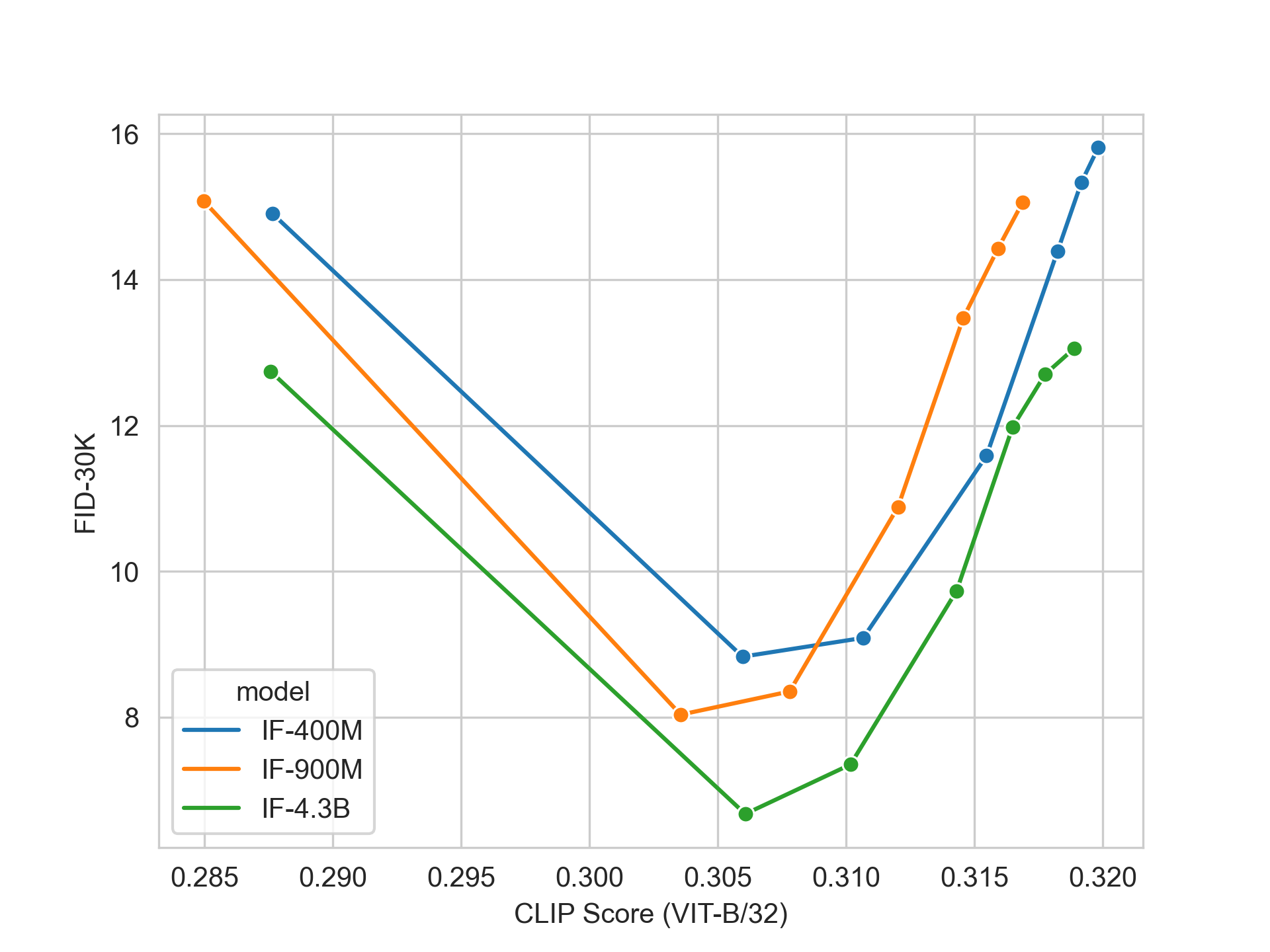
Example
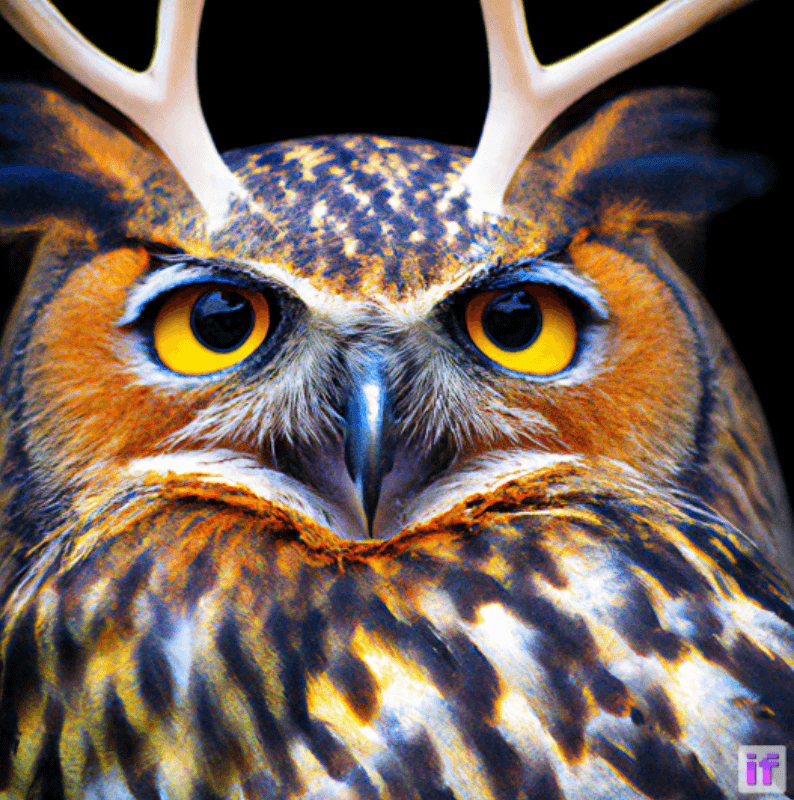
Usage
The model is released for research purposes. Any attempt to deploy the model in production requires not only that the LICENSE is followed but full liability over the person deploying the model.
Recommended Uses
According to the publishers, it is recommended to utilize the model for generating artistic imagery and incorporating it into design and other artistic processes. Additionally, the model holds potential for applications in educational or creative tools and is valuable for conducting research on generative models. One of the model's strengths lies in its ability to probe and gain understanding of the limitations and biases inherent in generative models, providing insights for further development and improvement.
Out-of-Scope Use
It is essential to adhere to responsible guidelines when utilizing generative models to avoid the generation of demeaning, dehumanizing, or otherwise harmful representations of individuals, their environments, cultures, religions, and so on. Intentionally promoting or propagating discriminatory content or harmful stereotypes should be strictly avoided. It is crucial to obtain consent before impersonating individuals, and generating or sharing sexual content without the consent of those who may come across it is unacceptable. Mis- and disinformation should be prevented, as well as representations of egregious violence and gore. Additionally, it is important to respect copyright and license agreements by refraining from sharing copyrighted or licensed material in violation of its terms of use, as well as abstaining from sharing content that alters copyrighted or licensed material in violation of its terms of use. By adhering to these principles, we can ensure ethical and responsible usage of generative models.
Limitations
While the model exhibits impressive capabilities, it is important to note that it does not attain flawless photorealism. It may produce outputs that fall short of perfect realism. Moreover, the model's training primarily focused on English captions, which implies that its performance may not be as robust when applied to other languages. It is worth considering that the training dataset, a subset of the extensive LAION-5B dataset, contains adult, violent, and sexual content. To address this concern to some extent, efforts have been made to mitigate the impact through specific measures, as outlined in the Training section.
Bias
IF was primarily trained on subsets of LAION-2B(en), which consists of images that are limited to English descriptions. Texts and images from communities and cultures that use other languages are likely to be insufficiently accounted for. This affects the overall output of the model, as white and western cultures are often set as the default. Further, the ability of the model to generate content with non-English prompts is significantly worse than with English-language prompts. IF mirrors and exacerbates biases to such a degree that viewer discretion must be advised irrespective of the input or its intent.
Sample Codes
Quick Start
pip install deepfloyd_if==1.0.2rc0 pip install xformers==0.0.16 pip install git+https://github.com/openai/CLIP.git --no-deps
Sample Code from diffusers import DiffusionPipeline and from diffusers.utils import pt_to_pil
import torch # stage 1 stage_1 = DiffusionPipeline.from_pretrained("DeepFloyd/IF-I-XL-v1.0", variant="fp16", torch_dtype=torch.float16) stage_1.enable_xformers_memory_efficient_attention() # remove line if torch.__version__ >= 2.0.0 stage_1.enable_model_cpu_offload() # stage 2 stage_2 = DiffusionPipeline.from_pretrained( "DeepFloyd/IF-II-L-v1.0", text_encoder=None, variant="fp16", torch_dtype=torch.float16 ) stage_2.enable_xformers_memory_efficient_attention() # remove line if torch.__version__ >= 2.0.0 stage_2.enable_model_cpu_offload() # stage 3 safety_modules = {"feature_extractor": stage_1.feature_extractor, "safety_checker": stage_1.safety_checker, "watermarker": stage_1.watermarker} stage_3 = DiffusionPipeline.from_pretrained("stabilityai/stable-diffusion-x4-upscaler", **safety_modules, torch_dtype=torch.float16) stage_3.enable_xformers_memory_efficient_attention() # remove line if torch.__version__ >= 2.0.0 stage_3.enable_model_cpu_offload()
Load the models into VRAM
from deepfloyd_if.modules import IFStageI, IFStageII, StableStageIII from deepfloyd_if.modules.t5 import T5Embedder device = 'cuda:0' if_I = IFStageI('IF-I-XL-v1.0', device=device) if_II = IFStageII('IF-II-L-v1.0', device=device) if_III = StableStageIII('stable-diffusion-x4-upscaler', device=device) t5 = T5Embedder(device="cpu")
Other LLMs
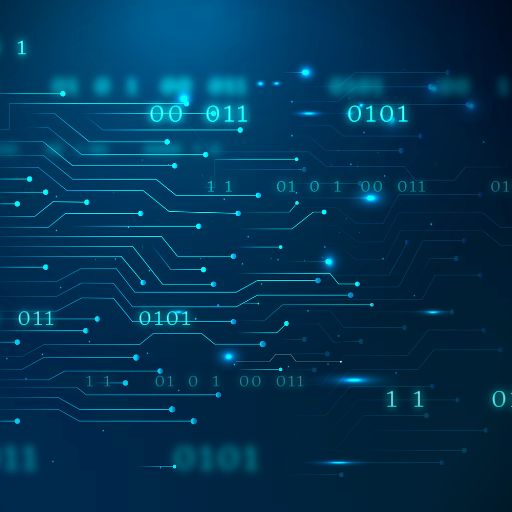
PFGM++
PFGM++ is a family of physics-inspired generative models that embeds trajectories for N dimensional data in N+D dimensional space using a simple scalar norm of additional variables.
Read More

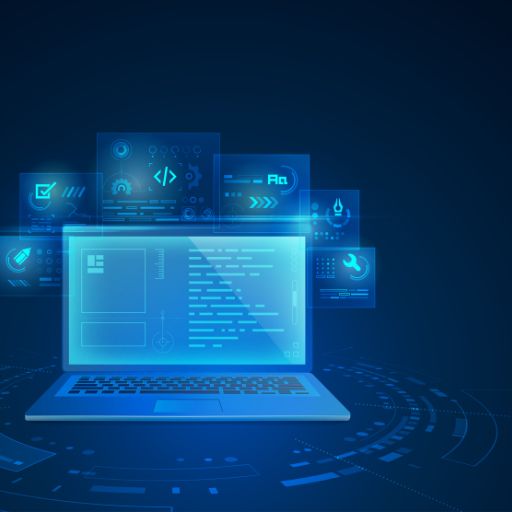
MDT-XL2
MDT proposes a mask latent modeling scheme for transformer-based DPMs to improve contextual and relation learning among semantics in an image.
Read More

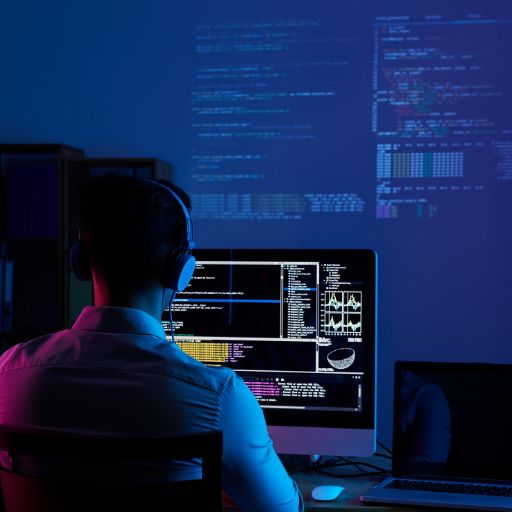
Stable Diffusion
An image synthesis model called Stable Diffusion produces high-quality results without the computational requirements of autoregressive transformers.
Read More
