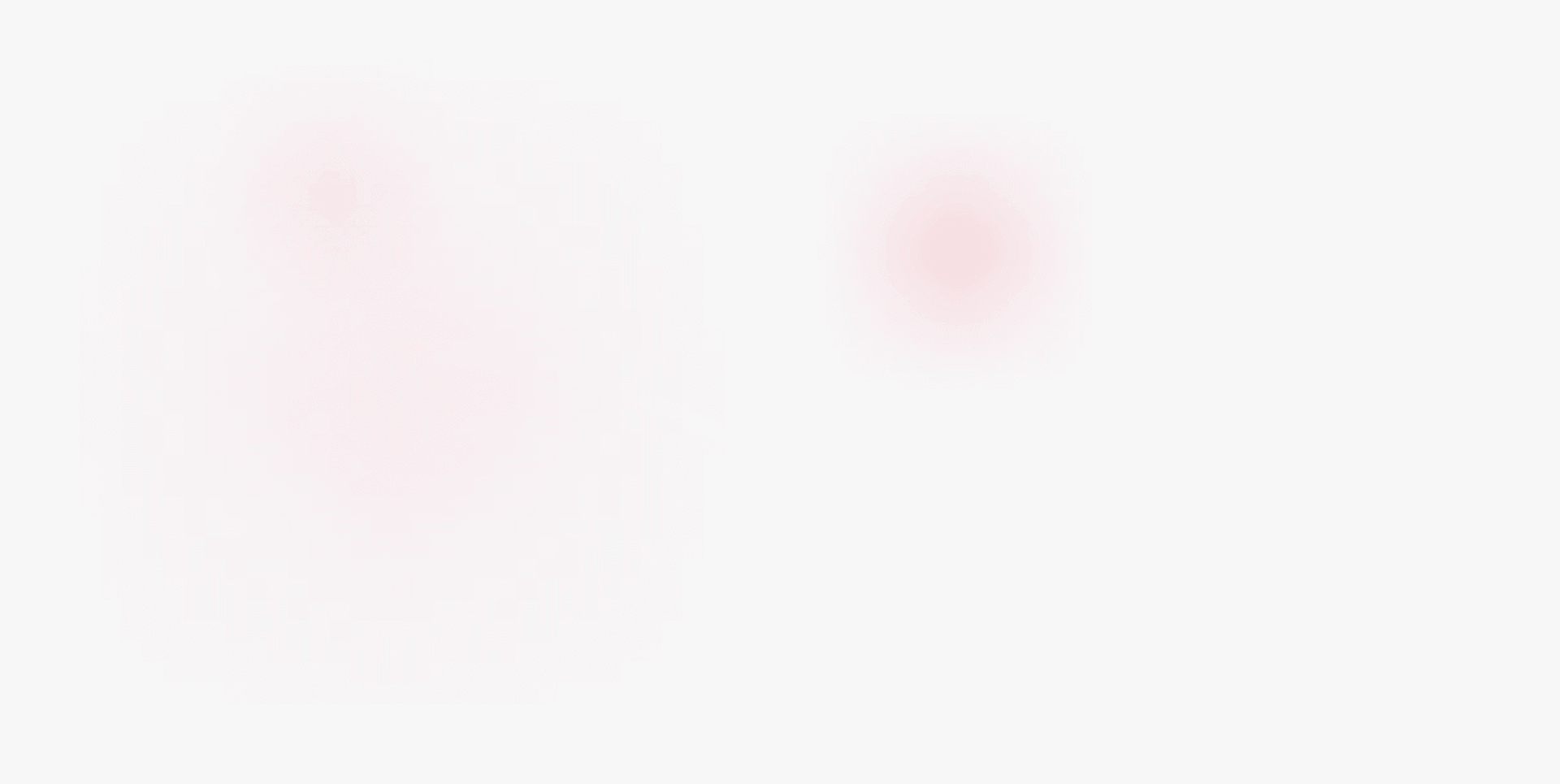
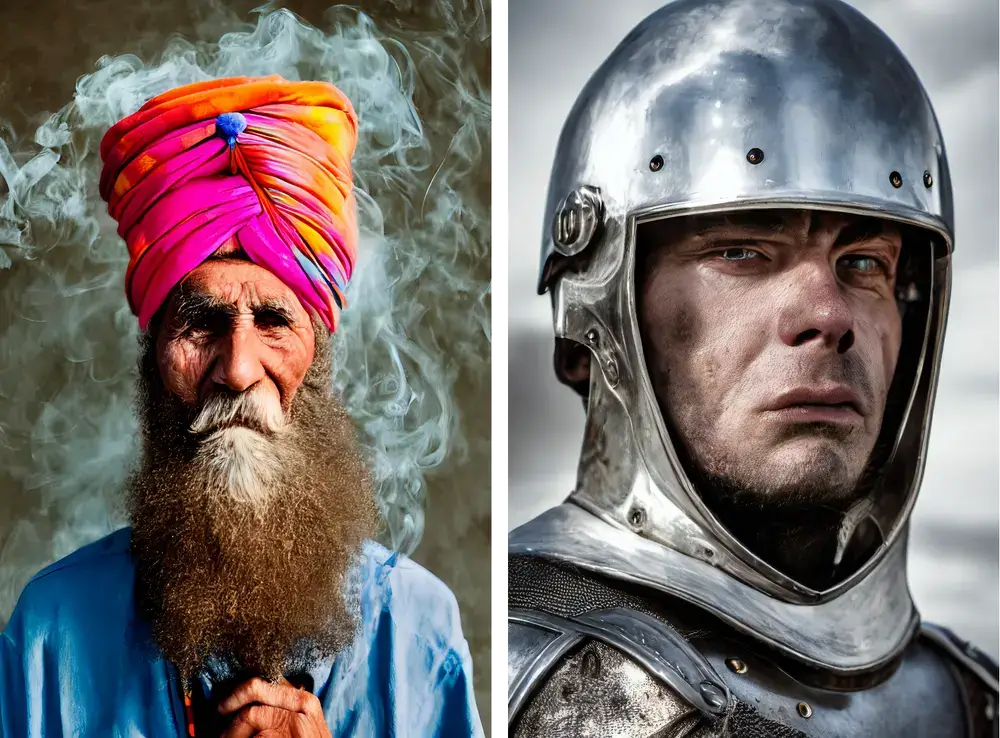
Text to Image,
Stable Diffusion V1
Stable Diffusion v1 is a text-to-image diffusion model that generates realistic images based on given text inputs. The Stable Diffusion v1.1 was trained on 237,000 steps at resolution 256x256 on laion2B-en, followed by 194,000 steps at resolution 512x512 on laion-high-resolution.
Model Card

100+ Technical Experts

50 Custom AI projects

4.8 Minimum Rating
Blockchain Success Starts here


-
About Model
-
Model Highlighter
-
Training Details
-
Results
-
Misuse
-
Sample Codes
-
Environmental Impact
-
Other LLMs
About Model
Stable Diffusion is an advanced model that converts text into highly realistic images. Using Latent Diffusion, you can generate photo-realistic images based on any given text input.
The training process for Stable-Diffusion-v1-1 involved 237,000 steps at a resolution of 256x256 on the dataset laion2B-en. Followed by an additional 194,000 steps at a resolution of 512x512 on the dataset lion-high-resolution, which consisted of 170 million examples from LAION-5B with a resolution of 1024x1024 or higher.
The model can generate and modify images based on text prompts. The Latent Diffusion Model uses a fixed, pretrained text encoder (CLIP ViT-L/14), as suggested in the Imagen paper.
Stable Diffusion V1 is under the CreativeML OpenRAIL M license, adapted from the work that BigScience and the RAIL Initiative are jointly carrying in responsible AI licensing.
Developed by: CompVis (Robin Rombach, Patrick Esser)
Model type: Diffusion-based text-to-image generation model
Language(s): English
Model Highlights
Stable Diffusion is based on Latent Diffusion, a process that can reduce the memory and compute complexity by applying the diffusion process over a lower dimensional latent space instead of using the actual pixel space. In latent Diffusion, the model is trained to generate latent (compressed) representations of the images.
Autoencoder
The VAE model comprises an encoder and a decoder. The encoder converts the image into a low-dimensional latent representation for the U-Net model. Conversely, the decoder transforms the latent representation back into an image. In latent diffusion training, the encoder obtains latent representations (latents) for the forward diffusion process, gradually adding more noise at each step. During inference, the denoised latents from the reverse diffusion process are converted into images using only the VAE decoder. Only the VAE decoder is required for inference.
U-Net
The U-Net architecture consists of an encoder and a decoder, both composed of ResNet blocks. The encoder compresses the image representation into a lower-resolution form, while the decoder decodes this lower-resolution representation back to the original higher-resolution image representation, aiming to reduce noise. Specifically, the U-Net output predicts the noise residual, which can be used to compute the denoised image representation.
Text-encoder
The text-encoder is tasked with converting the input prompt, such as "An apple with a cap," into an embedding space that the U-Net can interpret. Typically, it employs a straightforward transformer-based encoder that maps a sequence of input tokens to a sequence of latent text embeddings. In the case of Stable Diffusion, it draws inspiration from Imagen and does not train the text-encoder during the training process. Instead, it utilizes an already trained text encoder called CLIPTextModel from CLIP.
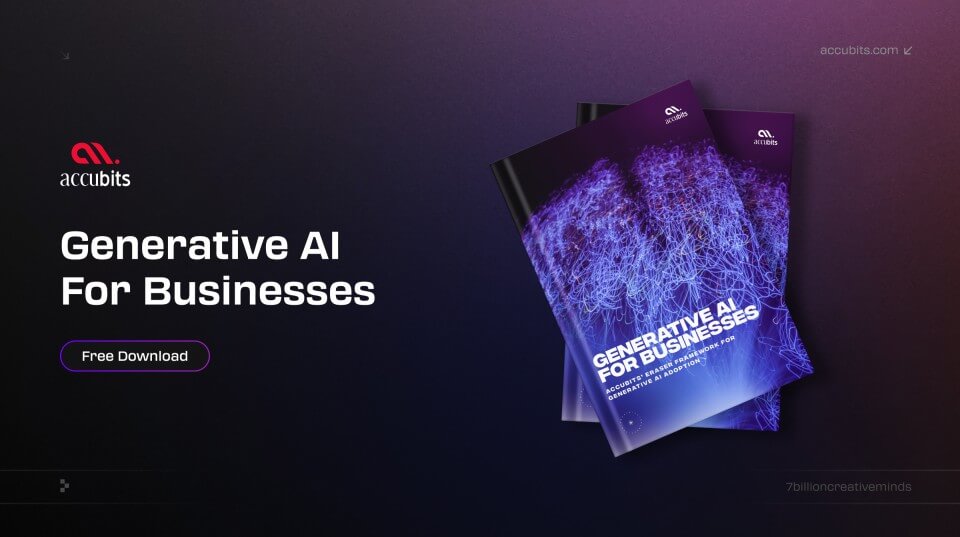
Training Details
Training
The model developers used LAION-2B (en) and its subsets to train the model. During training, Images are encoded through an encoder, which turns images into latent representations. The autoencoder uses a relative downsampling factor of 8 and maps images of shape H x W x 3 to latents of shape H/f x W/f x 4. Text prompts are encoded through a ViT-L/14 text encoder. The non-pooled output of the text encoder is fed into the UNet backbone of the latent diffusion model via cross-attention. The loss is a reconstruction objective between the noise added to the latent and the prediction made by the UNet.
A total of 32 x 8 x A100 GPUs were used for the training. AdamW optimizer and Gradient Accumulations of 2 were used for the training data batched with 32 x 8 x 2 x 4 = 2048 batches. Learning rate was set to warmup to 0.0001 for 10,000 steps and then kept constant
Results
Evaluations with different classifier-free guidance scales (1.5, 2.0, 3.0, 4.0, 5.0, 6.0, 7.0, 8.0) and 50 PLMS sampling steps show the relative improvements of the checkpoints. Evaluated using 50 PLMS steps and 10000 random prompts from the COCO2017 validation set, evaluated at 512x512 resolution. Not optimized for FID scores.
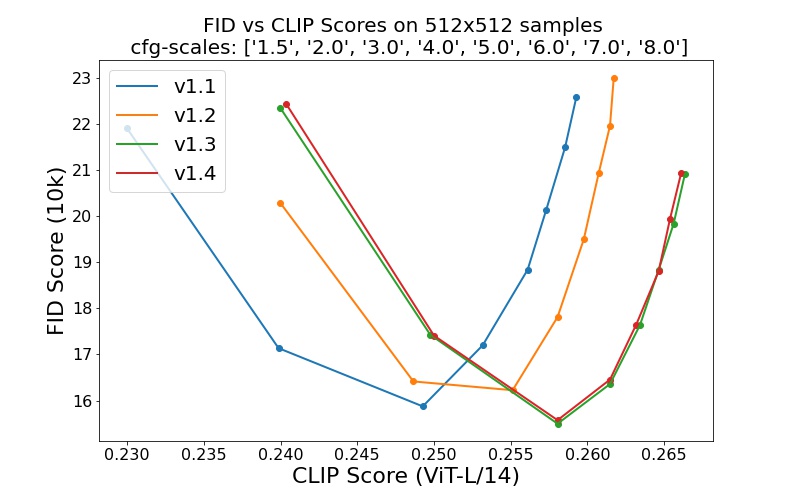
Examples
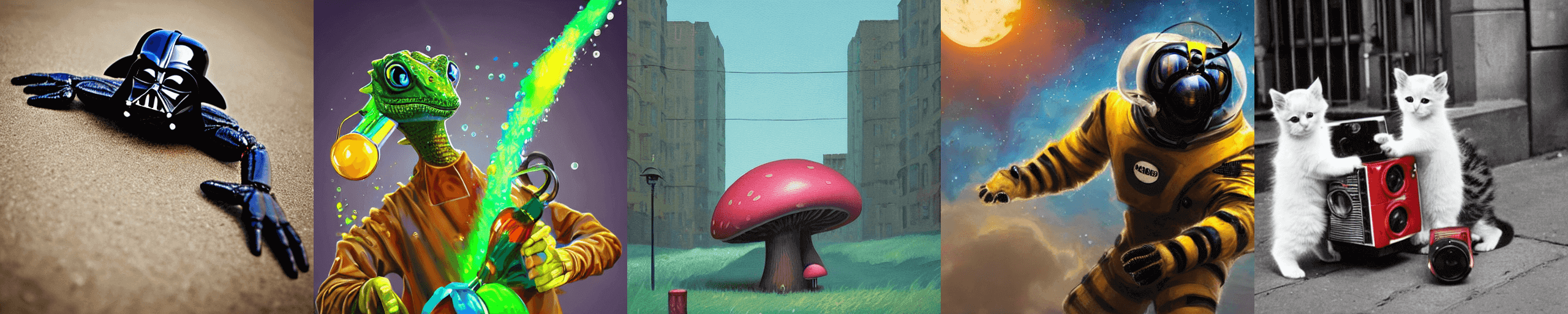
Usage
The model is primarily designed for research purposes and offers potential applications in various areas and tasks. These include ensuring the safe deployment of models that have the capability to generate harmful content, investigating and comprehending the limitations and biases inherent in generative models, utilizing the model for generating artworks and supporting design and other artistic processes, exploring its potential applications in educational or creative tools, and conducting research specifically focused on generative models. However, it is important to note that there are certain uses that are excluded or not recommended for this model. These excluded uses are described below to provide clarity and ensure responsible utilization of the model.
Out-of-Scope Use
The model should not be utilized with the intention of generating or spreading images that contribute to hostile or alienating environments for individuals. This includes avoiding the generation of images that are expected to be disturbing, distressing, or offensive to people, as well as refraining from creating content that reinforces historical or present-day stereotypes. Responsible usage of the model should prioritize the avoidance of such outputs.
The model was not trained to be factual or true representations of people or events, and therefore using the model to generate such content is out-of-scope for the abilities of this model.
Malicious Use
Misusing the model by generating content that inflicts cruelty upon individuals is strongly discouraged. This encompasses a range of actions, including but not limited to generating demeaning, dehumanizing, or otherwise harmful representations of people, their environments, cultures, and religions. It also includes intentionally promoting or spreading discriminatory content or harmful stereotypes, impersonating individuals without their consent, creating and sharing non-consensual sexual content, disseminating mis- and disinformation, depicting extreme violence and gore, sharing copyrighted or licensed material in violation of its terms of use, and distributing content that alters copyrighted or licensed material without authorization. It is essential to adhere to these guidelines to ensure responsible and respectful use of the model.
Limitations
It is important to note that the model has certain limitations and may not achieve perfect photorealism. It may struggle to render legible text and may not perform well on more complex tasks involving compositionality, such as generating an image of a "red cube on top of a blue sphere." Additionally, the generation of faces and people in general may not always be accurate.
Furthermore, it is worth mentioning that the model's training primarily focused on English captions. As a result, its performance may not be as strong when used with other languages. The autoencoding aspect of the model is also lossy, meaning that there may be some loss of information during the encoding and decoding process.
Lastly, it is crucial to recognize that the model was trained on a dataset (LAION-5B) that includes adult material. Therefore, using the model in a product or public setting requires additional safety mechanisms and considerations to ensure appropriateness and compliance with ethical standards.
Bias
While the capabilities of image generation models are impressive, they can also reinforce or exacerbate social biases. Stable Diffusion v1 was trained on subsets of LAION-2B(en), which consists of images that are primarily limited to English descriptions. Texts and images from communities and cultures that use other languages are likely to be insufficiently accounted for. This affects the overall output of the model, as white and western cultures are often set as the default. Further, the ability of the model to generate content with non-English prompts is significantly worse than with English-language prompts.
Sample Codes
Create and activate suitable conda environment
conda env create -f environment.yaml conda activate ldm
Update an existing latent diffusion environment
conda install pytorch torchvision -c pytorch pip install transformers==4.19.2 diffusers invisible-watermark pip install -e .
Sample Code from diffusers import StableDiffusionPipeline
import torch model_id = "runwayml/stable-diffusion-v1-5" pipe = StableDiffusionPipeline.from_pretrained(model_id, torch_dtype=torch.float16) pipe = pipe.to("cuda") prompt = "a photo of an astronaut riding a horse on mars" image = pipe(prompt).images[0] image.save("astronaut_rides_horse.png")
Environmental Impact
An estimation of CO2 emissions for Stable Diffusion v1 was conducted using the Machine Learning Impact calculator. Considering the hardware type (A100 PCIe 40GB), total hours of usage (150,000), cloud provider (AWS), and compute region (US-east), the carbon impact was estimated to be approximately 11,250 kg CO2 eq. This estimation is based on factors such as power consumption, time, and the carbon produced according to the location of the power grid.
Other LLMs
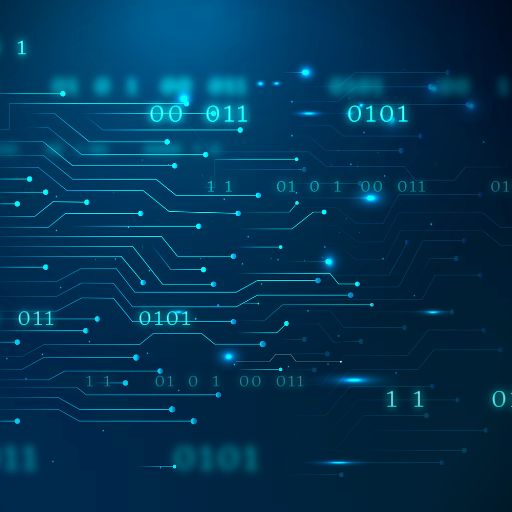
PFGM++
PFGM++ is a family of physics-inspired generative models that embeds trajectories for N dimensional data in N+D dimensional space using a simple scalar norm of additional variables.
Read More

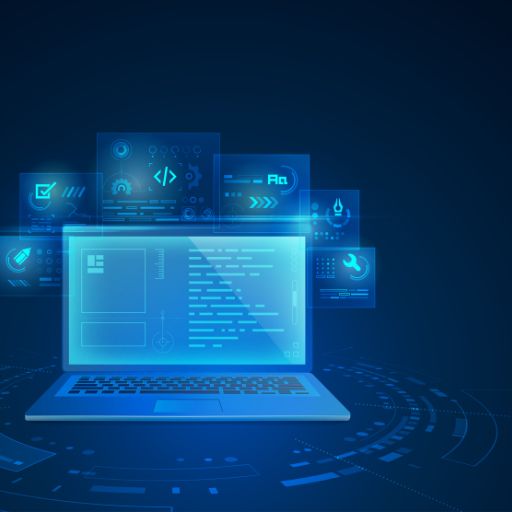
MDT-XL2
MDT proposes a mask latent modeling scheme for transformer-based DPMs to improve contextual and relation learning among semantics in an image.
Read More

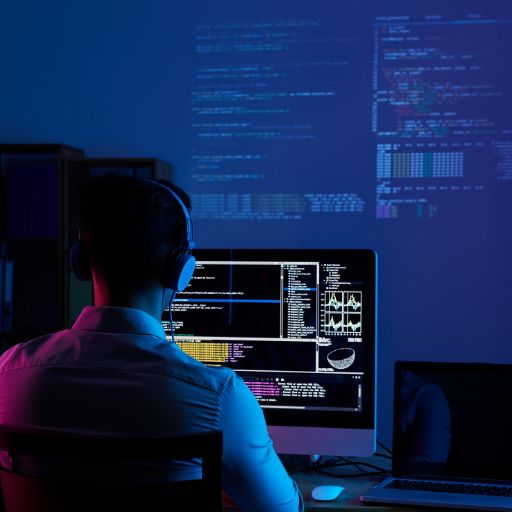
Stable Diffusion
An image synthesis model called Stable Diffusion produces high-quality results without the computational requirements of autoregressive transformers.
Read More
