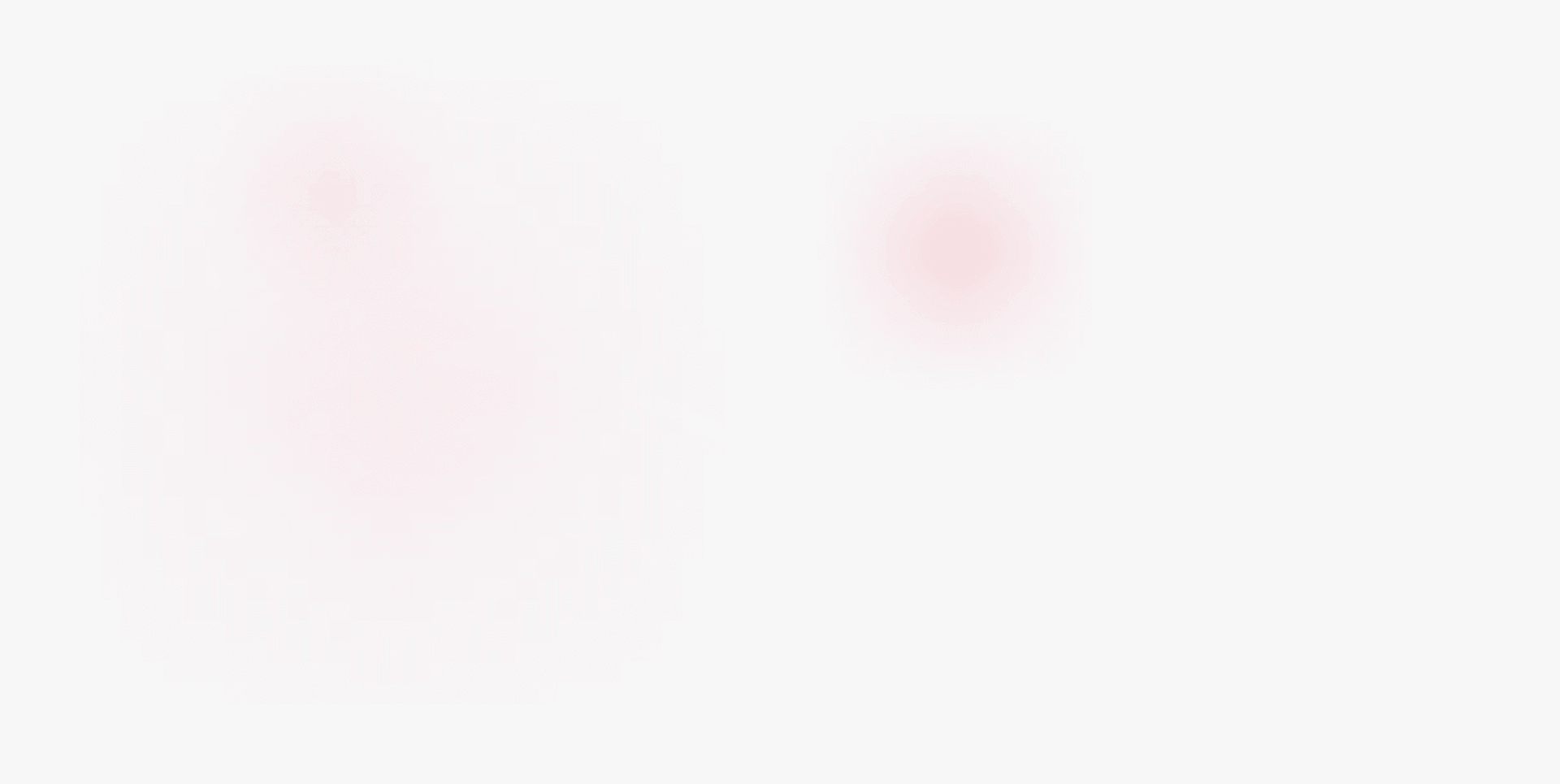
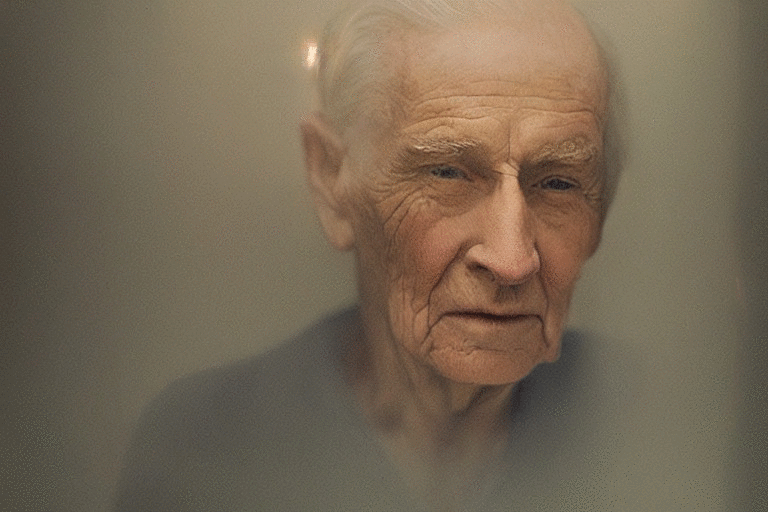
Text to Image
Stable Diffusion V2
Stable Diffusion v2 refers to a specific configuration of the model architecture that uses a downsampling-factor 8 autoencoder with an 865M UNet and OpenCLIP ViT-H/14 text encoder for the diffusion model. The SD 2-v model produces 768x768 px outputs.
Model Card

100+ Technical Experts

50 Custom AI projects

4.8 Minimum Rating
Blockchain Success Starts here


-
About Model
-
Training Details
-
Misuse
-
Sample Codes
-
Environmental Impact
-
Results
-
Other LLMs
About Model
Stable Diffusion is an advanced model that converts text into highly realistic images. Using Latent Diffusion, you can generate photo-realistic images based on any given text input. Stable Diffusion v2 refers to a specific configuration of the model architecture that uses a downsampling-factor 8 autoencoder with an 865M UNet and OpenCLIP ViT-H/14 text encoder for the diffusion model. The SD 2-v model produces 768x768 px outputs.
Developed by: Robin Rombach, Patrick Esser
Model type: Diffusion-based text-to-image generation model
Language(s): English
Model Highlights
Stable Diffusion is based on Latent Diffusion, a process that can reduce the memory and compute complexity by applying the diffusion process over a lower dimensional latent space instead of using the actual pixel space. In latent Diffusion, the model is trained to generate latent (compressed) representations of the images.
Autoencoder
The VAE model comprises an encoder and a decoder. The encoder converts the image into a low-dimensional latent representation for the U-Net model. Conversely, the decoder transforms the latent representation back into an image. In latent diffusion training, the encoder obtains latent representations (latents) for the forward diffusion process, gradually adding more noise at each step. During inference, the denoised latents from the reverse diffusion process are converted into images using only the VAE decoder. Only the VAE decoder is required for inference.
U-Net
The U-Net architecture consists of an encoder and a decoder, both composed of ResNet blocks. The encoder compresses the image representation into a lower-resolution form, while the decoder decodes this lower-resolution representation back to the original higher-resolution image representation, aiming to reduce noise. Specifically, the U-Net output predicts the noise residual, which can be used to compute the denoised image representation.
Text-encoder
The text-encoder is tasked with converting the input prompt, such as "An apple with a cap," into an embedding space that the U-Net can interpret. Typically, it employs a straightforward transformer-based encoder that maps a sequence of input tokens to a sequence of latent text embeddings. In the case of Stable Diffusion, it draws inspiration from Imagen and does not train the text-encoder during the training process. Instead, it utilizes an already trained text encoder called CLIPTextModel from CLIP.
Training Details
The model developers used LAION-5B and subsets for training the model. The training data is further filtered using LAION's NSFW detector, with a "p_unsafe" score of 0.1 (conservative).
During training, the image encoding process involves utilizing an encoder that transforms images into latent representations. The autoencoder employs a relative downsampling factor of 8, converting images of shape H x W x 3 to latents with a shape of H/f x W/f x 4. On the other hand, text prompts are encoded using the OpenCLIP-ViT/H text-encoder.
The output of the text encoder is then fed into the UNet backbone of the latent diffusion model through cross-attention. This allows for the integration of textual information into the image generation process.
To ensure the quality of the generated images, a reconstruction objective is employed as the loss function. This objective measures the similarity between the noise added to the latent representation and the prediction made by the UNet. This loss helps guide the training process and improve the fidelity of the generated outputs.
Checkpoint | Details |
512-base-ema.ckpt | dimension550k steps at resolution 256x256 on a subset of LAION-5B filtered for explicit pornographic material, using the LAION-NSFW classifier with punsafe=0.1 and an aesthetic score >= 4.5. 850k steps at resolution 512x512 on the same dataset with resolution >= 512x512. |
768-v-ema.ckpt | Resumed from 512-base-ema.ckpt and trained for 150k steps using a v-objective on the same dataset. Resumed for another 140k steps on a 768x768 subset of our dataset. |
512-depth-ema.ckpt | Resumed from 512-base-ema.ckpt and finetuned for 200k steps. Added an extra input channel to process the (relative) depth prediction produced by MiDaS (dpt_hybrid) which is used as an additional conditioning. The additional input channels of the U-Net which process this extra information were zero-initialized. |
512-inpainting-ema.ckpt | Resumed from 512-base-ema.ckpt and trained for another 200k steps. Follows the mask-generation strategy presented in LAMA which, in combination with the latent VAE representations of the masked image, are used as an additional conditioning. The additional input channels of the U-Net which process this extra information were zero-initialized. The same strategy was used to train the 1.5-inpainting checkpoint. |
x4-upscaling-ema.ckpt | Trained for 1.25M steps on a 10M subset of LAION containing images >2048x2048. The model was trained on crops of size 512x512 and is a text-guided latent upscaling diffusion model. In addition to the textual input, it receives a noise_level as an input parameter, which can be used to add noise to the low-resolution input according to a predefined diffusion schedule. |
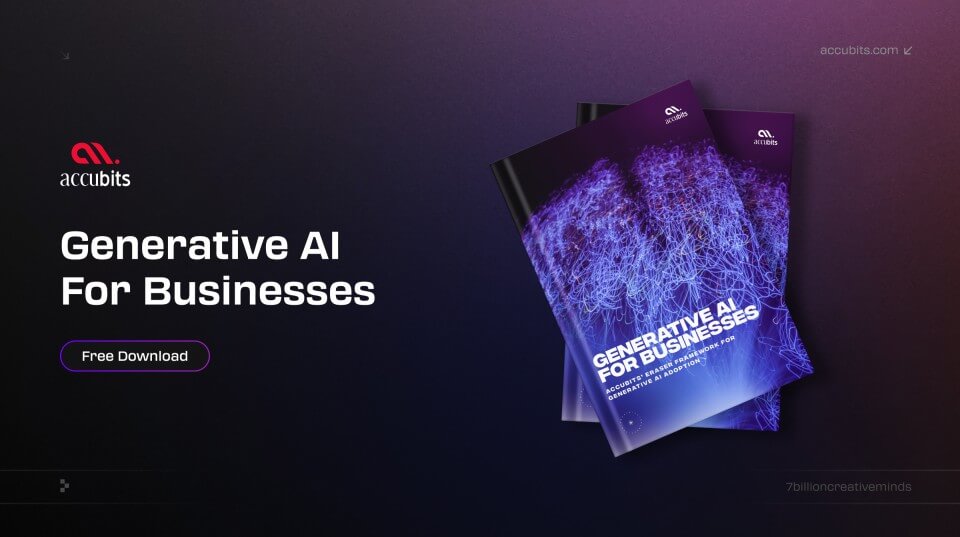
Usage
The model should not be used to intentionally create or disseminate images that create hostile or alienating environments for people. This includes generating images that people would foreseeably find disturbing, distressing, or offensive; or content that propagates historical or current stereotypes.
Out-of-Scope Use
The model was not trained to be factual or true representations of people or events, and therefore using the model to generate such content is out-of-scope for the abilities of this model.
Misuse and Malicious Use
Misusing the model to generate content that is cruel towards individuals goes against the intended purpose of this model. Such misuse includes various actions, such as generating demeaning, dehumanizing, or otherwise harmful representations of people, their environments, cultures, religions, and more. It also involves intentionally promoting or propagating discriminatory content or harmful stereotypes, impersonating individuals without their consent, creating and sharing sexual content without the consent of the individuals who may see it, spreading mis- and disinformation, creating representations of extreme violence and gore, sharing copyrighted or licensed material in violation of its terms of use, and distributing content that is an unauthorized alteration of copyrighted or licensed material. It is essential to refrain from engaging in these activities and use the model responsibly and ethically.
Limitations
The model has certain limitations that are important to consider. Firstly, it does not achieve perfect photorealism, meaning that the generated images may not appear indistinguishable from real photos. Additionally, the model struggles with rendering legible text, so the generated text may not be easily readable.
Furthermore, the model may face difficulties in handling more complex tasks involving compositionality. For instance, it may struggle to accurately generate an image corresponding to a prompt like "A red cube on top of a blue sphere." In terms of generating faces and people, there is a possibility that the model may not generate them properly, resulting in less accurate representations.
It is worth noting that the model's training primarily focused on English captions, which means its performance may not be as strong when used with other languages. The autoencoding part of the model is lossy, indicating that there may be some loss of information during the encoding and decoding process, affecting the fidelity of the generated outputs.
Lastly, the model was trained on a subset of the larger LAION-5B dataset, which includes adult, violent, and sexual content. To address this concern, the dataset was filtered using LAION's NFSW (Not Safe For Work) detector, aiming to partially mitigate the presence of such content in the training data.
Bias
While the capabilities of image generation models are impressive, they can also reinforce or exacerbate social biases. Stable Diffusion was primarily trained on subsets of LAION-2B(en), which consists of images that are limited to English descriptions. Texts and images from communities and cultures that use other languages are likely to be insufficiently accounted for. This affects the overall output of the model, as white and western cultures are often set as the default. Further, the ability of the model to generate content with non-English prompts is significantly worse than with English-language prompts. Stable Diffusion v2 mirrors and exacerbates biases to such a degree that viewer discretion must be advised irrespective of the input or its intent.
Sample Codes
Running the pipeline
from diffusers import StableDiffusionPipeline, EulerDiscreteScheduler model_id = "stabilityai/stable-diffusion-2" # Use the Euler scheduler here instead scheduler = EulerDiscreteScheduler.from_pretrained(model_id, subfolder="scheduler") pipe = StableDiffusionPipeline.from_pretrained(model_id, scheduler=scheduler, torch_dtype=torch.float16) pipe = pipe.to("cuda") prompt = "a photo of an astronaut riding a horse on mars" image = pipe(prompt).images[0] image.save("astronaut_rides_horse.png")
Update an existing latent diffusion environment
conda install pytorch==1.12.1 torchvision==0.13.1 -c pytorch pip install transformers==4.19.2 diffusers invisible-watermark pip install -e .
For more efficiency and speed on GPUs, instal the xformers library.
export CUDA_HOME=/usr/local/cuda-11.4 conda install -c nvidia/label/cuda-11.4.0 cuda-nvcc conda install -c conda-forge gcc conda install -c conda-forge gxx_linux-64==9.5.0
Environmental Impact
Taking into account the hardware type (A100 PCIe 40GB), the total hours of usage (200,000), the cloud provider (AWS), and the compute region (US-east), the estimated carbon emissions amount to approximately 15,000 kg CO2 eq. This estimation considers factors such as power consumption, time, and the carbon produced based on the location of the power grid.
Results
Stable Diffusion v2 refers to a specific configuration of the model architecture that uses a downsampling-factor 8 autoencoder with an 865M UNet and OpenCLIP ViT-H/14 text encoder for the diffusion model. The SD 2-v model produces 768x768 px outputs. Evaluations with different classifier-free guidance scales (1.5, 2.0, 3.0, 4.0, 5.0, 6.0, 7.0, 8.0) and 50 DDIM sampling steps show the relative improvements of the checkpoints:
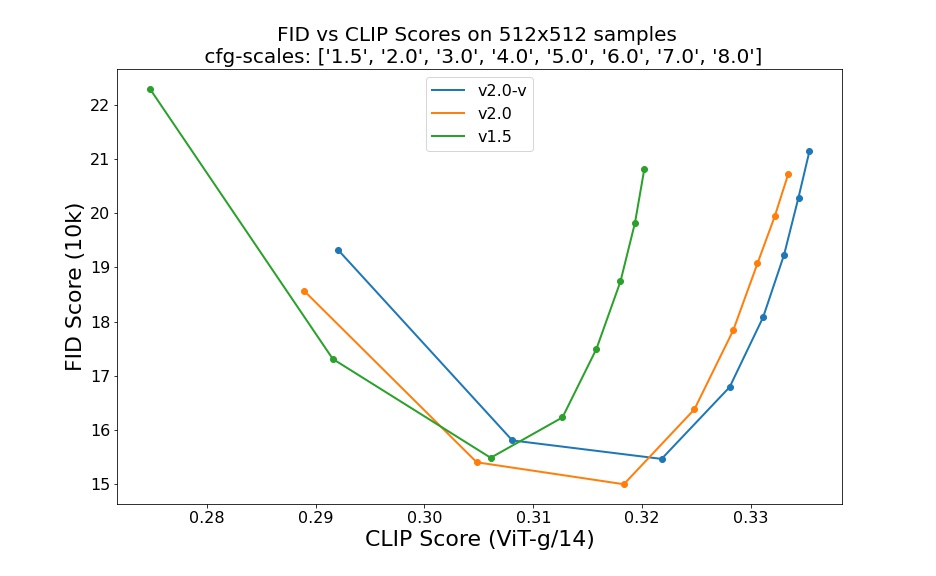
Examples
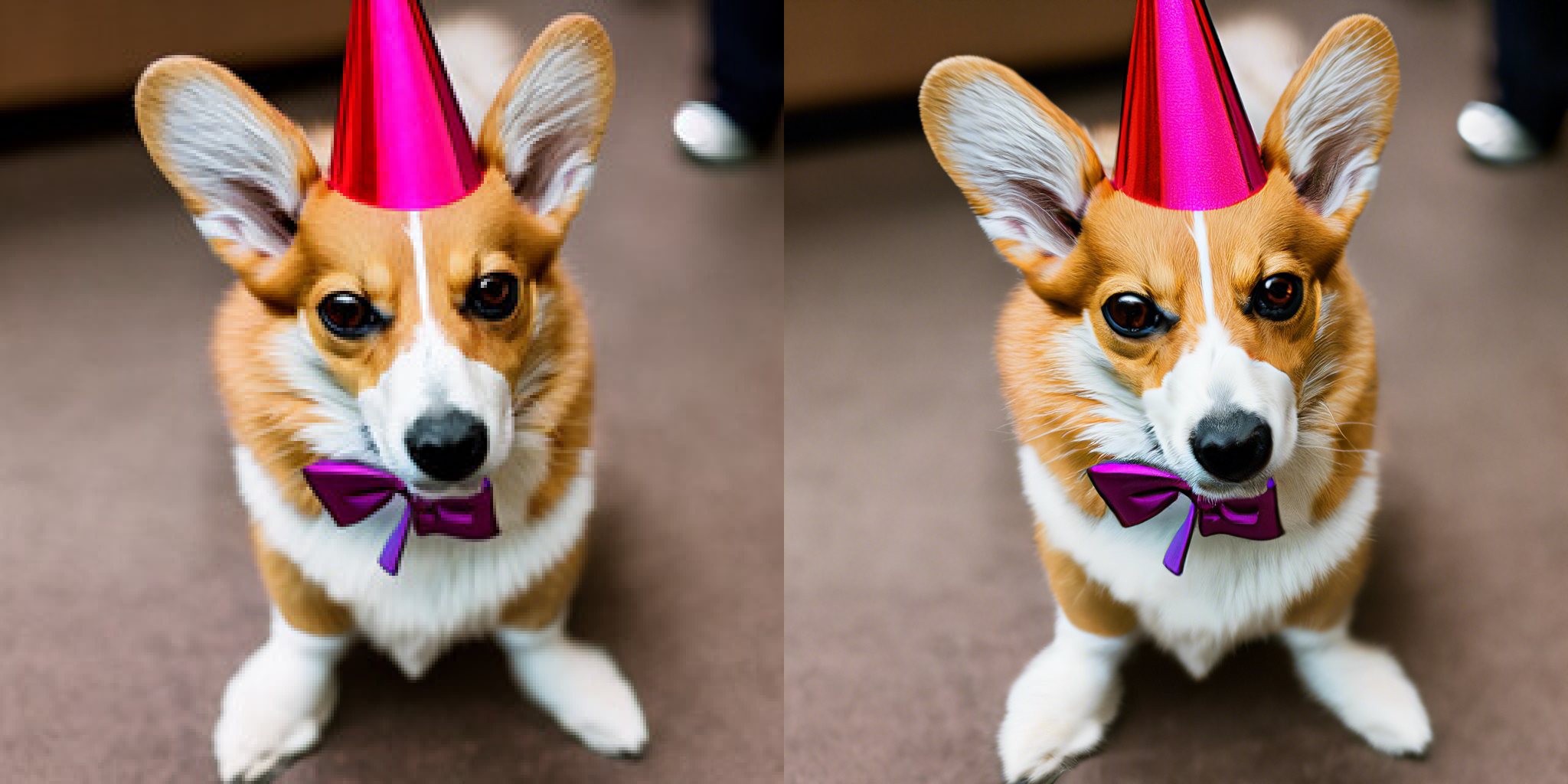
Other LLMs
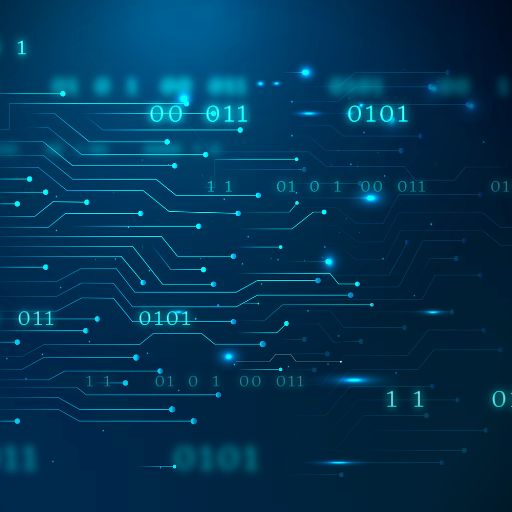
PFGM++
PFGM++ is a family of physics-inspired generative models that embeds trajectories for N dimensional data in N+D dimensional space using a simple scalar norm of additional variables.
Read More

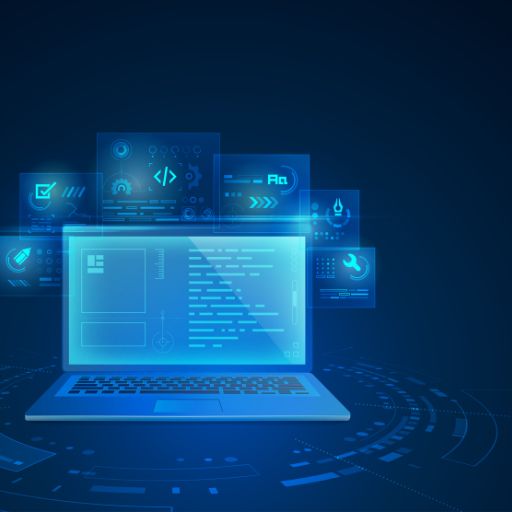
MDT-XL2
MDT proposes a mask latent modeling scheme for transformer-based DPMs to improve contextual and relation learning among semantics in an image.
Read More

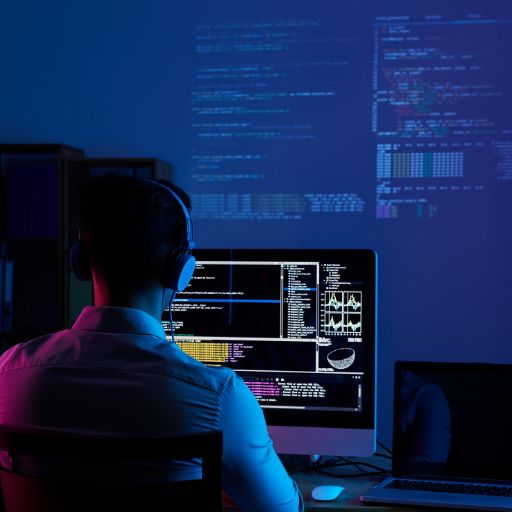
Stable Diffusion
An image synthesis model called Stable Diffusion produces high-quality results without the computational requirements of autoregressive transformers.
Read More
